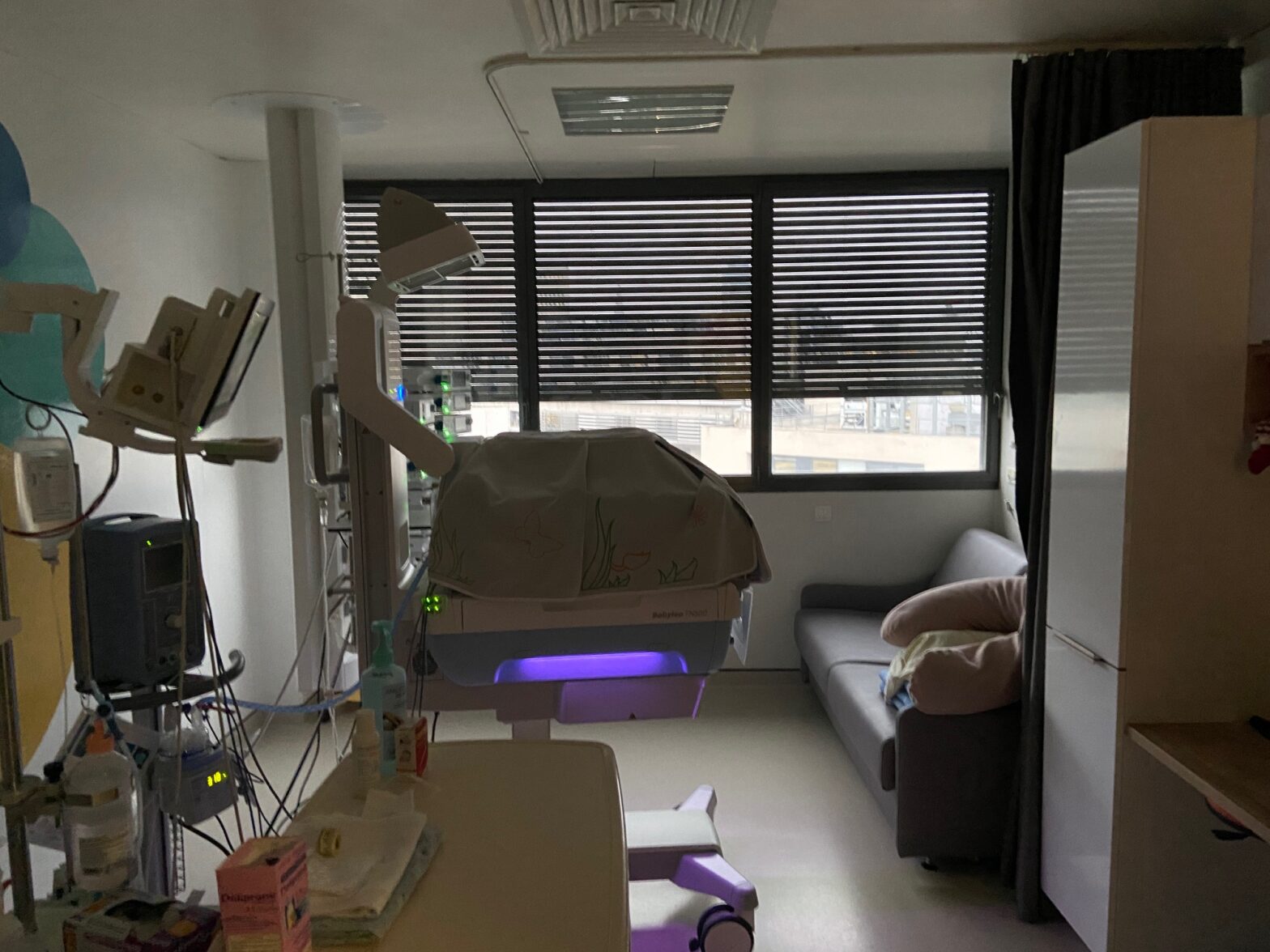
Machine listening in a neonatal intensive care unit
Communications dans un congrès
Auteurs : Modan Tailleur, Vincent Lostanlen, Jean-Philippe Rivière, Pierre Aumond.
Conférence : Workshop on Detection and Classification of Acoustic Scenes and Events (DCASE)
Date de publication : 2024
Computational environmental audio analysisEdge computingMachine learning methodsPrivacy