UPCOMING EVENTS

OTHER NEWS
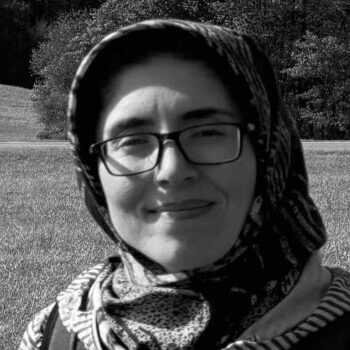
Introducing: Reyhaneh Abbasi
Reyhaneh is working on the generation of mouse ultrasonic vocalizations, with applications to animal behavior research.
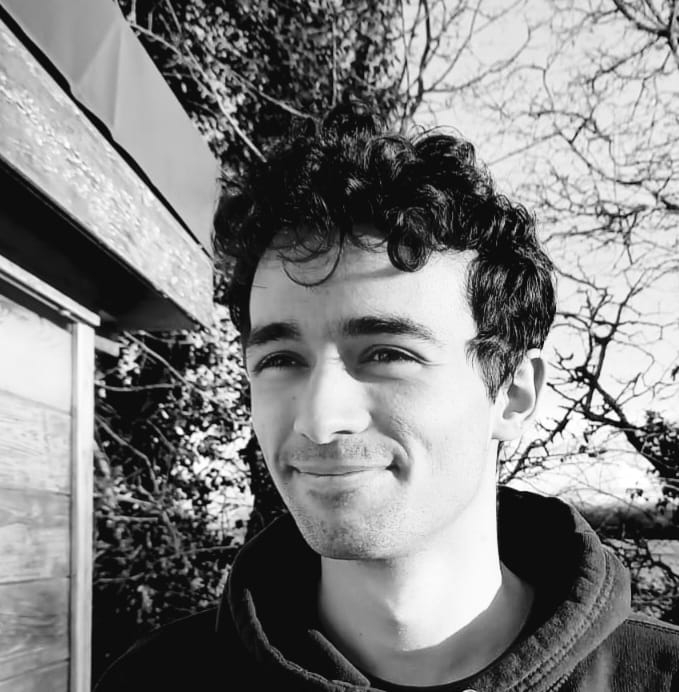
Introducing: Matthieu Carreau
Matthieu is working on audio signal processing algorithms that can run on autonomous sensors subject to intermitent power supply. He is a PhD student, advised by Vincent Lostanlen, Pierre-Emmanuel Hladik, and Sébastien Faucou.
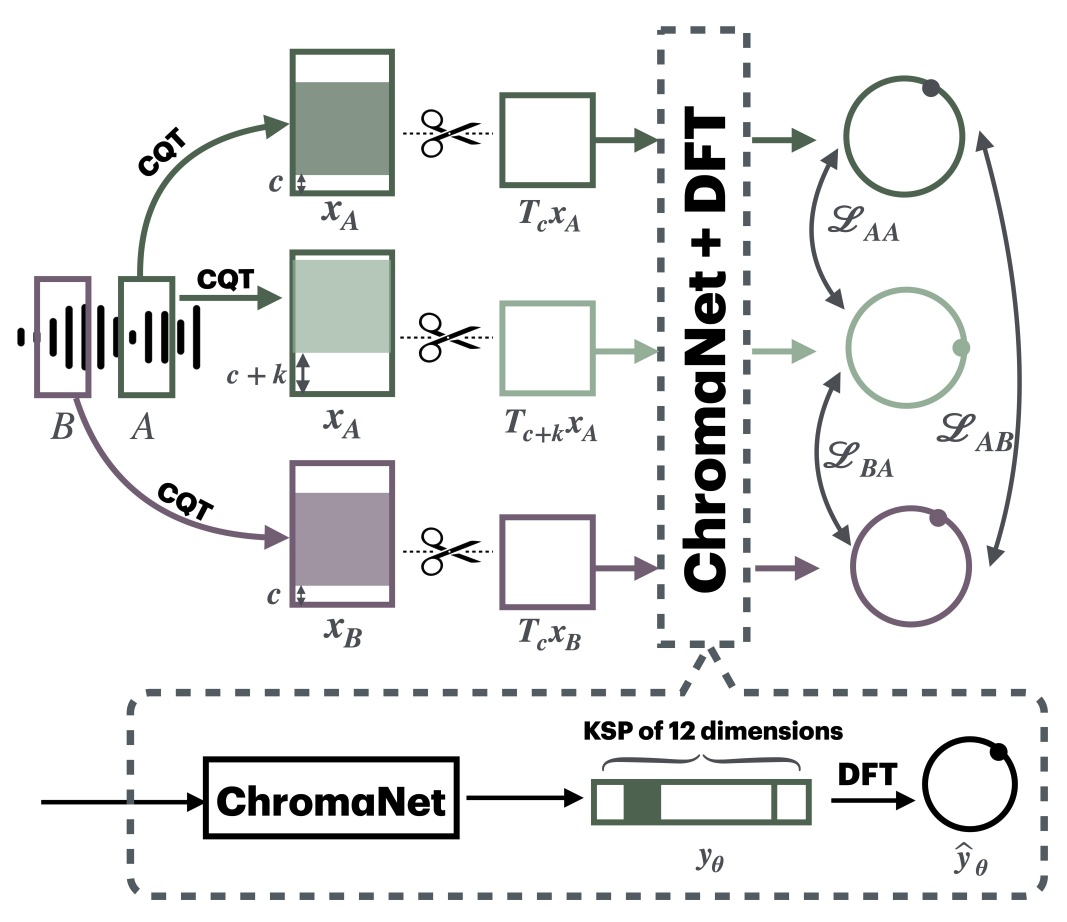
STONE: Self-supervised tonality estimator @ ISMIR
Although deep neural networks can estimate the key of a musical piece, their supervision incurs a massive annotation effort. Against this shortcoming, we present STONE, the first self-supervised tonality estimator. The architecture behind STONE, named ChromaNet, is a convnet with octave equivalence which outputs a “key signature profile” (KSP) of 12 structured logits. First, we train ChromaNet to regress artificial pitch transpositions between any two unlabeled musical excerpts from the same audio track, as measured as cross-power spectral density (CPSD) within the circle of fifths (CoF). We observe that this self-supervised pretext task leads KSP to correlate with tonal key signature. Based on this observation, we extend STONE to output a structured KSP of 24 logits, and introduce supervision so as to disambiguate major versus minor keys sharing the same key signature. Applying different amounts of supervision yields semi-supervised and fully supervised tonality estimators: i.e., Semi-TONEs and Sup-TONEs. We evaluate these estimators on FMAK, a new dataset of 5489 real-world musical recordings with expert annotation of 24 major and minor keys. We find that Semi-TONE matches the classification accuracy of Sup-TONE with reduced supervision and outperforms it with equal supervision.
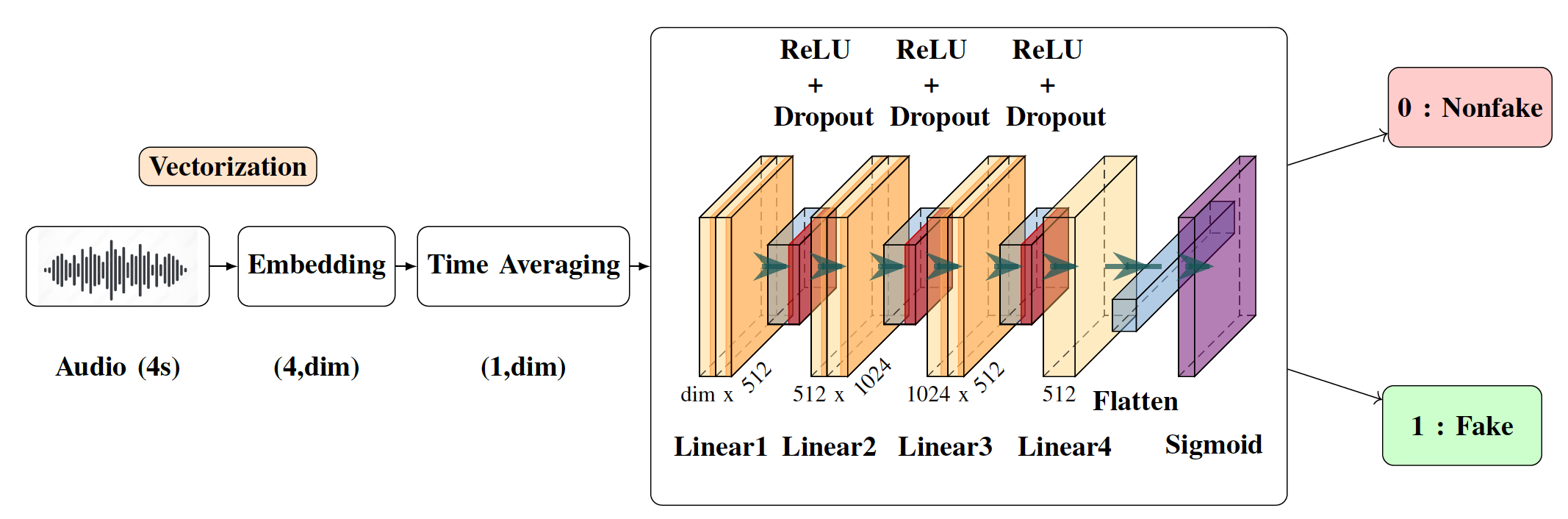
Detection of Deepfake Environmental Audio @ EUSIPCO
With the ever-rising quality of deep generative models,
it is increasingly important to be able to discern whether the
audio data at hand have been recorded or synthesized. Although
the detection of fake speech signals has been studied extensively,
this is not the case for the detection of fake environmental audio.
We propose a simple and efficient pipeline for detecting fake
environmental sounds based on the CLAP audio embedding. We
evaluate this detector using audio data from the 2023 DCASE
challenge task on Foley sound synthesis.
Our experiments show that fake sounds generated by 44 stateof-
the-art synthesizers can be detected on average with 98% accuracy.
We show that using an audio embedding trained specifically
on environmental audio is beneficial over a standard VGGish
one as it provides a 10% increase in detection performance. The
sounds misclassified by the detector were tested in an experiment
on human listeners who showed modest accuracy with nonfake
sounds, suggesting there may be unexploited audible features.
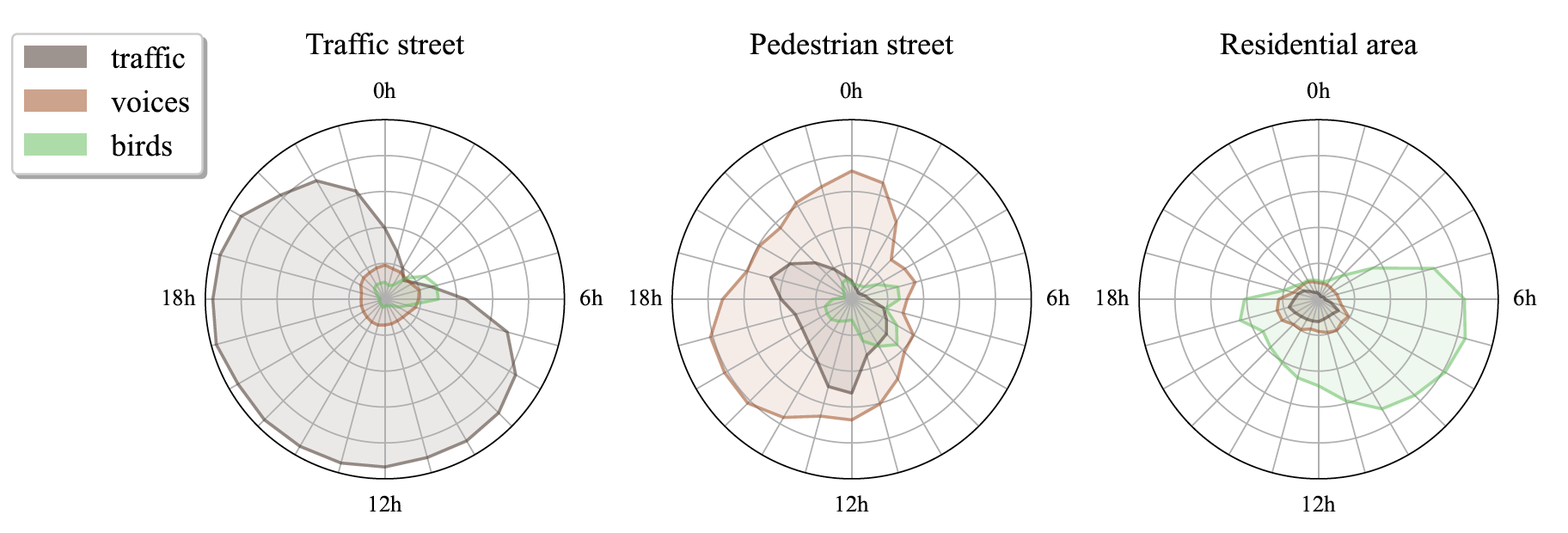
Sound source classification for soundscape analysis using fast third-octave bands data from an urban acoustic sensor network @ JASA
The exploration of the soundscape relies strongly on the characterization of the sound sources in the sound environment. Novel sound source classifiers, called pre-trained audio neural networks (PANNs), are capable of predicting the presence of more than 500 diverse sound sources. Nevertheless, PANNs models use fine Mel spectro-temporal representations as input, whereas sensors of an urban noise monitoring network often record fast third-octaves data, which have significantly lower spectro-temporal resolution. In a previous study, we developed a transcoder to transform fast third-octaves into the fine Mel spectro-temporal representation used as input of PANNs. In this paper, we demonstrate that employing PANNs with fast third-octaves data, processed through this transcoder, does not strongly degrade the classifier’s performance in predicting the perceived time of presence of sound sources. Through a qualitative analysis of a large-scale fast third-octave dataset, we also illustrate the potential of this tool in opening new perspectives and applications for monitoring the soundscapes of cities.
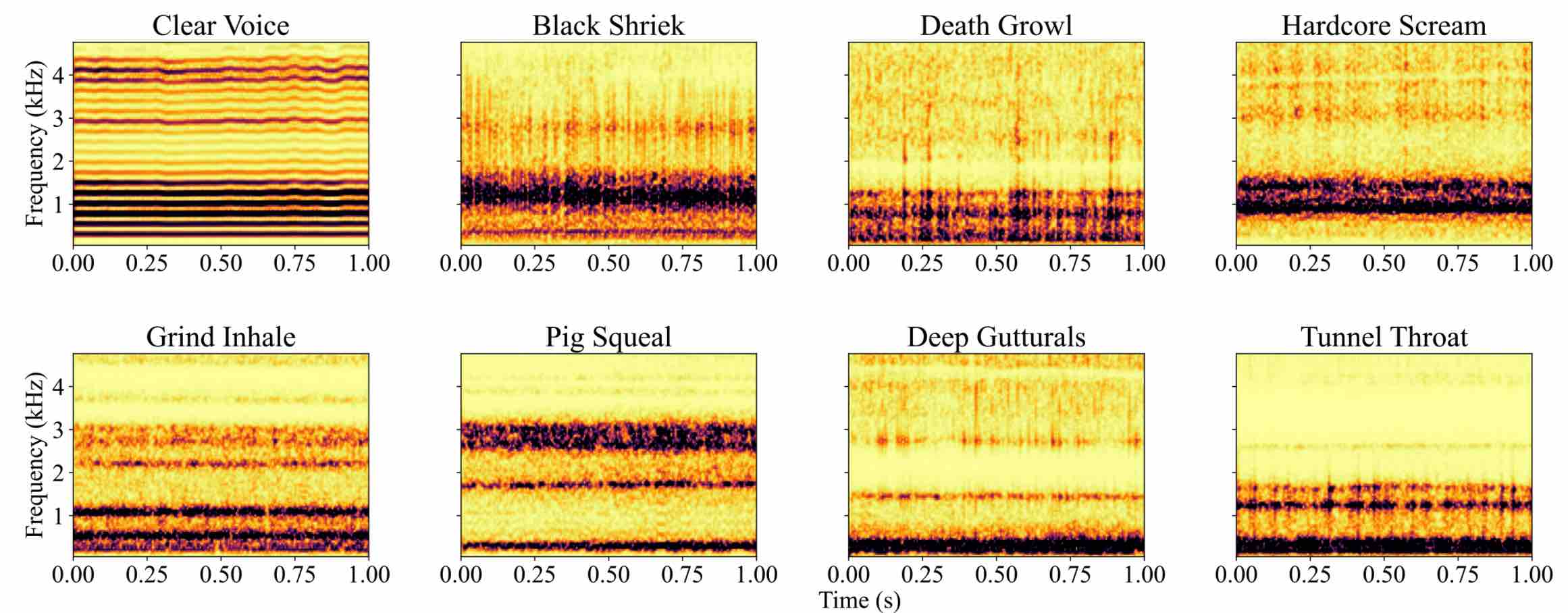
EMVD dataset: a dataset of extreme vocal distortion techniques used in heavy metal @ CBMI
In this paper, we introduce the Extreme Metal Vocals Dataset, which comprises a collection of recordings of extreme vocal techniques performed within the realm of heavy metal music. The dataset consists of 760 audio excerpts of 1 second to 30 seconds long, totaling about 100 min of audio material, roughly composed of 60 minutes of distorted voices and 40 minutes of clear voice recordings. These vocal recordings are from 27 different singers and are provided without accompanying musical instruments or post-processing effects. The distortion taxonomy within this dataset encompasses four distinct distortion techniques and three vocal effects, all performed in different pitch ranges. Performance of a state-of-the-art deep learning model is evaluated for two different classification tasks related to vocal techniques, demonstrating the potential of this resource for the audio processing community.
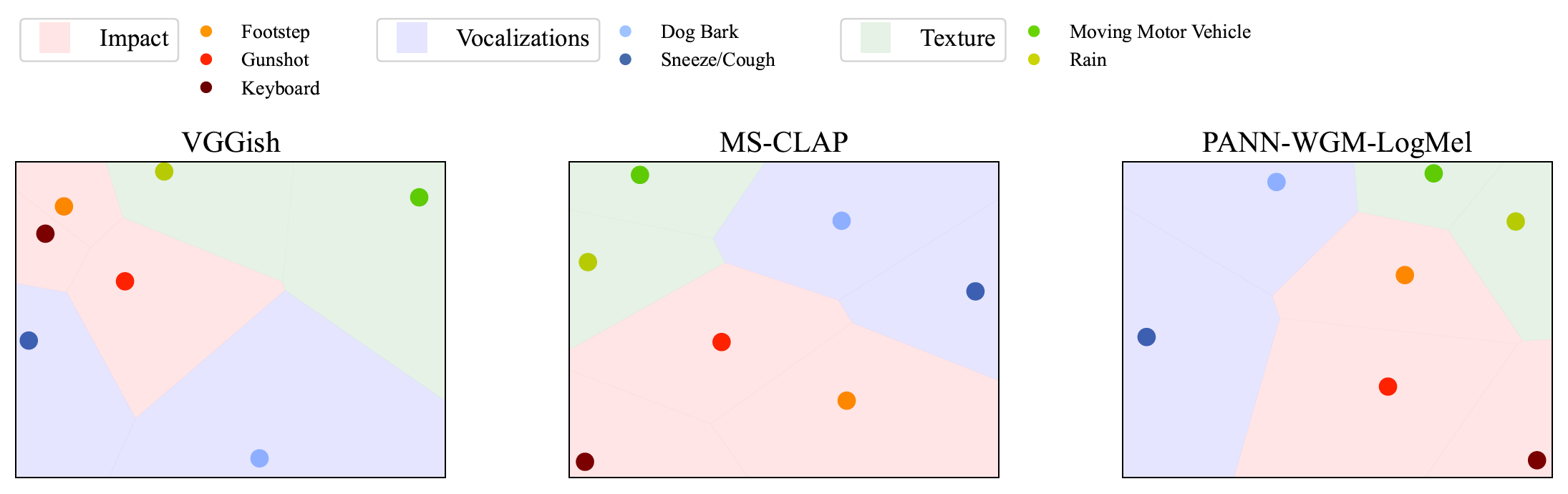
Correlation of Fréchet Audio Distance With Human Perception of Environmental Audio Is Embedding Dependent @ EUSIPCO
This paper explores whether considering alternative domain-specific embeddings to calculate the Frechet Audio Dis- tance (FAD) metric can help the FAD to correlate better with perceptual ratings of environmental sounds. We used embeddings from VGGish, PANNs, MS-CLAP, L-CLAP, and MERT, which are tailored for either music or environmental sound evaluation. The FAD scores were calculated for sounds from the DCASE 2023 Task 7 dataset. Using perceptual data from the same task, we find that PANNs-WGM-LogMel produces the best correlation between FAD scores and perceptual ratings of both audio quality and perceived fit with a Spearman correlation higher than 0.5. We also find that music-specific embeddings resulted in significantly lower results. Interestingly, VGGish, the embedding used for the original Frechet calculation, yielded a correlation below 0.1. These results underscore the critical importance of the choice of embedding for the FAD metric design.
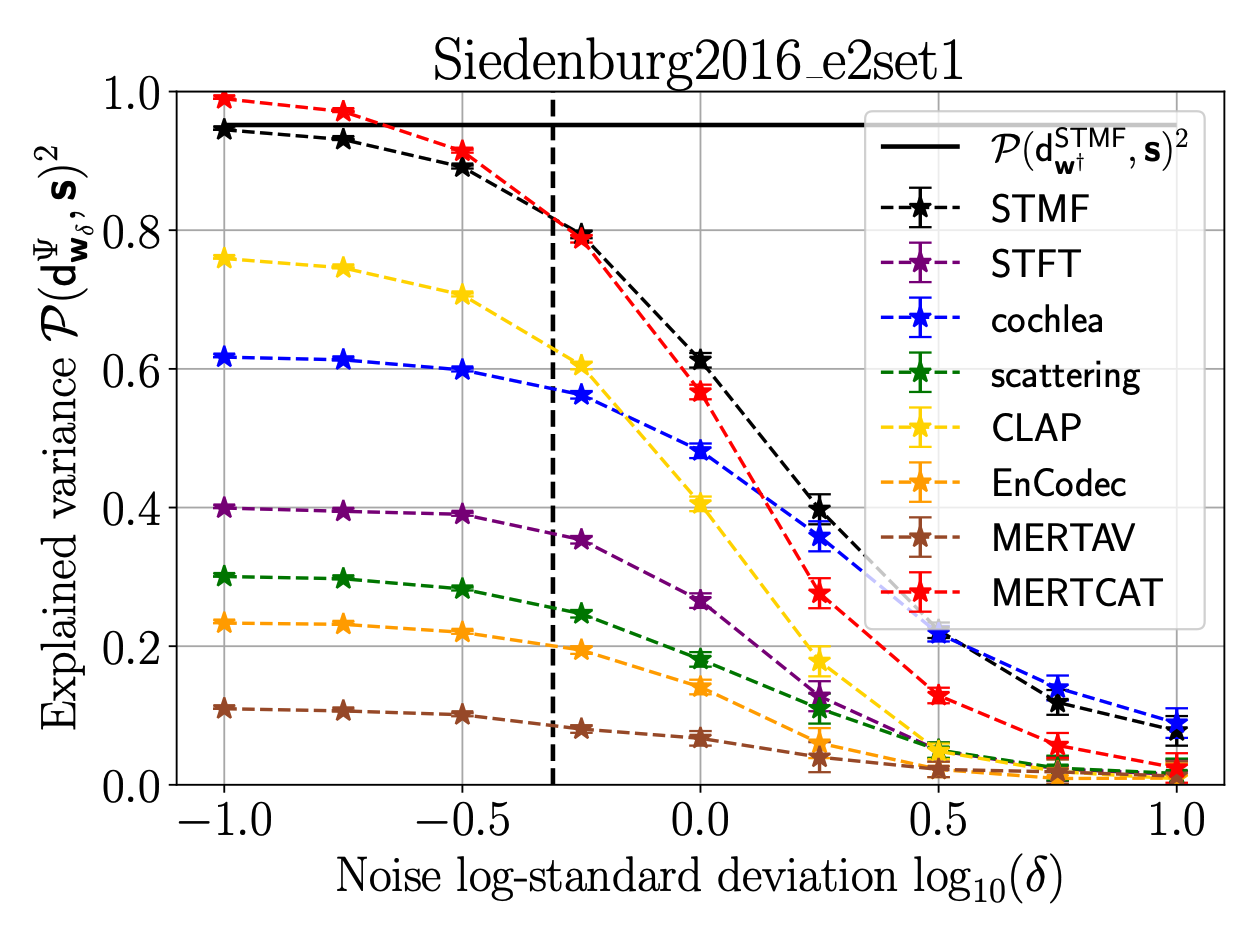
On the Robustness of Musical Timbre Perception Models: From Perceptual to Learned Approaches @ EUSIPCO
Timbre, encompassing an intricate set of acoustic cues, is key to identify sound sources, and especially to discriminate musical instruments and playing styles. Psychoacoustic studies focusing on timbre deploy massive efforts to explain human timbre perception. To uncover the acoustic substrates of timbre perceived dissimilarity, a recent work leveraged metric learning strategies on different perceptual representations and performed a meta-analysis of seventeen dissimilarity rated musical audio datasets. By learning salient patterns in very high-dimensional representations, metric learning accounts for a reasonably large part of the variance in human ratings. The present work shows that combining the most recent deep audio embeddings with a metric learning approach makes it possible to explain almost all the variance in human dissimilarity ratings. Furthermore, the robustness of the learning procedure against simulated human rating variability is thoroughly investigated. Intensive numerical experiments support the explanatory power and robustness against degraded dissimilarity ratings of the learning metric strategy using deep embeddings.
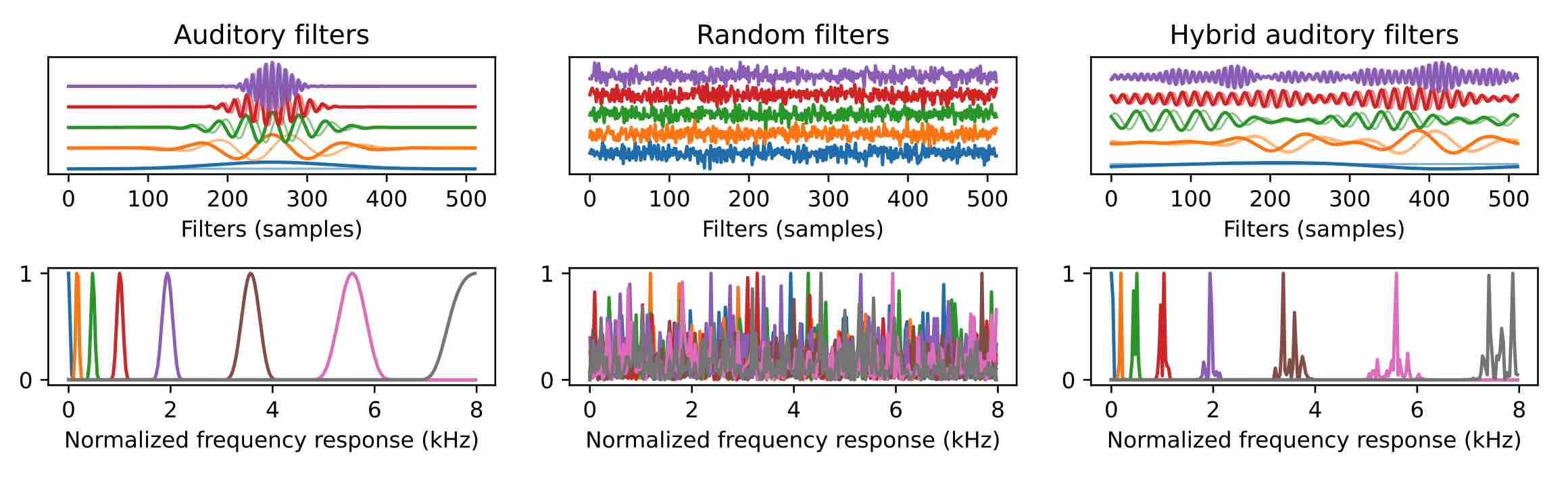
Hold Me Tight: Stable Encoder–Decoder Design for Speech Enhancement
Convolutional layers with 1-D filters are often used as frontend to encode audio signals. Unlike fixed time-frequency representations, they can adapt to the local characteristics of input data. However, 1-D filters on raw audio are hard to train and often suffer from instabilities. In this paper, we address these problems with hybrid solutions, i.e., combining theory-driven and datadriven approaches. First, we preprocess the audio signals via a auditory filterbank, guaranteeing good frequency localization for the learned encoder. Second, we use results from frame theory to define an unsupervised learning objective that encourages energy conservation and perfect reconstruction. Third, we adapt mixed compressed spectral norms as learning objectives to the encoder coefficients. Using these solutions in a low-complexity encoder-mask-decoder model significantly improves the perceptual evaluation of speech quality (PESQ) in speech enhancement.
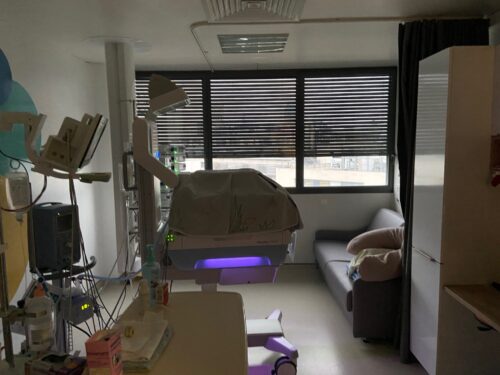
Machine Listening in a Neonatal Intensive Care Unit @ DCASE
Oxygenators, alarm devices, and footsteps are some of the most common sound sources in a hospital. Detecting them has scientific value for environmental psychology but comes with challenges of its own: namely, privacy preservation and limited labeled data. In this paper, we address these two challenges via a combination of edge computing and cloud computing. For privacy preservation, we have designed an acoustic sensor which computes third-octave spectrograms on the fly instead of recording audio waveforms. For sample-efficient machine learning, we have repurposed a pretrained audio neural network (PANN) via spectral transcoding and label space adaptation. A small-scale study in a neonatological intensive care unit (NICU) confirms that the time series of detected events align with another modality of measurement: i.e., electronic badges for parents and healthcare professionals. Hence, this paper demonstrates the feasibility of polyphonic machine listening in a hospital ward while guaranteeing privacy by design.
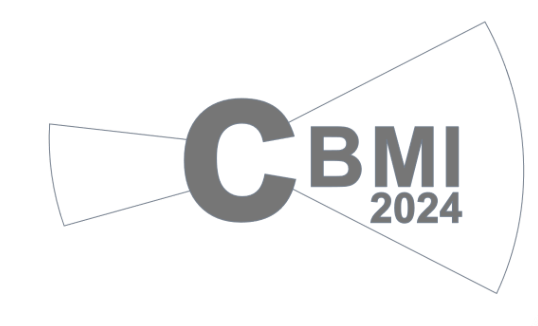
Content-Based Indexing for Audio and Music: From Analysis to Synthesis
Audio has long been a key component of multimedia research. As far as indexing is concerned, the research and industrial context has changed drastically in the last 20 years or so. Today, applications of audio indexing range from karaoke applications to singing voice synthesis and creative audio design. This special session aims at bringing together researchers that aim at proposing new tools or paradigms to investigate audio and music processing in the context of indexation and corpus-based generation.
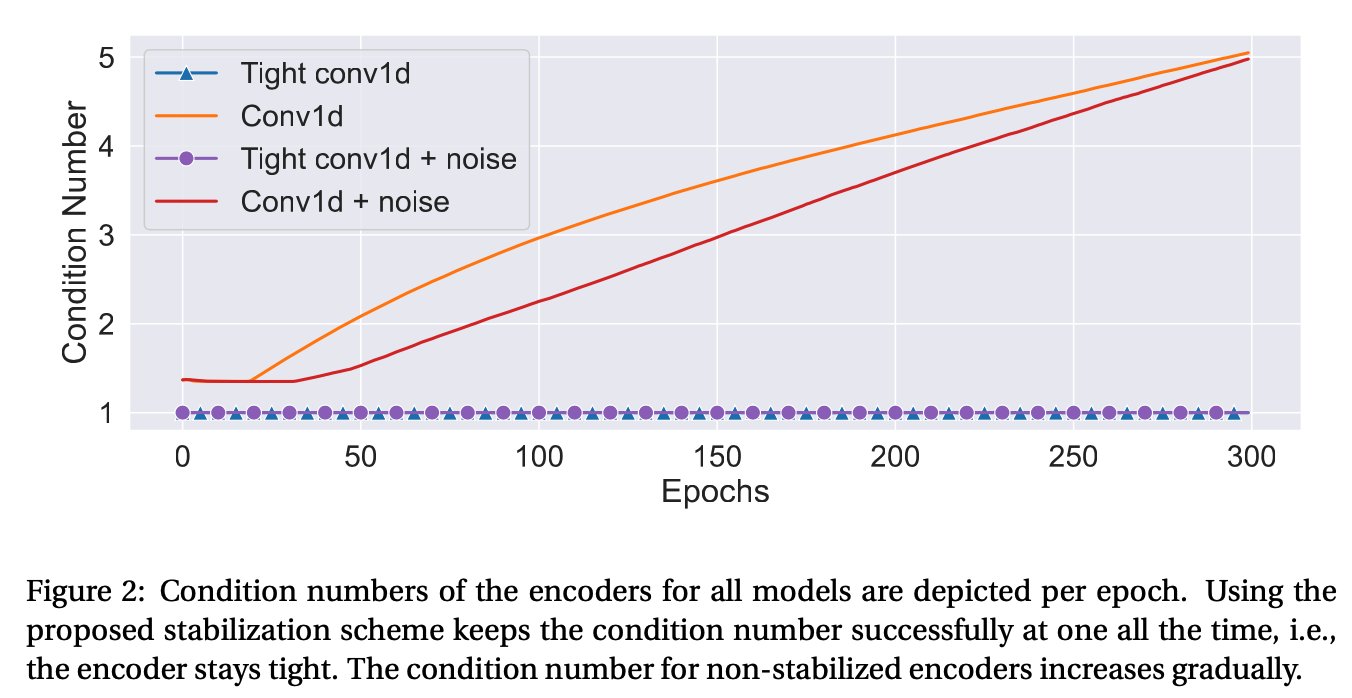
Trainable signal encoders that are robust against noise @ Inter-Noise
Within the deep learning paradigm, finite impulse response (FIR) filters are often used to encode audio signals, yielding flexible and adaptive feature representations. We show that a stabilization of FIR filterbanks with fixed filter lengths (convolutional layers with 1-D filters)leads to encoders that are optimally robust against noise and can be inverted with perfect reconstruction by their transposes. To maintain their flexibility as regular neural network layers, we implement the stabilization via a computationally efficient regularizing term in the objective function of the learning problem. In this way, the encoder keeps its expressive power and is optimally stable and noise-robust throughout the whole learning procedure. We show in a denoising task where noise is present in the input and in the encoder representation, that the proposed stabilization of the trainable filterbank encoder is decisive for increasing the signal-to-noise ratio of the denoised signals significantly compared to a model with a naively trained encoder.
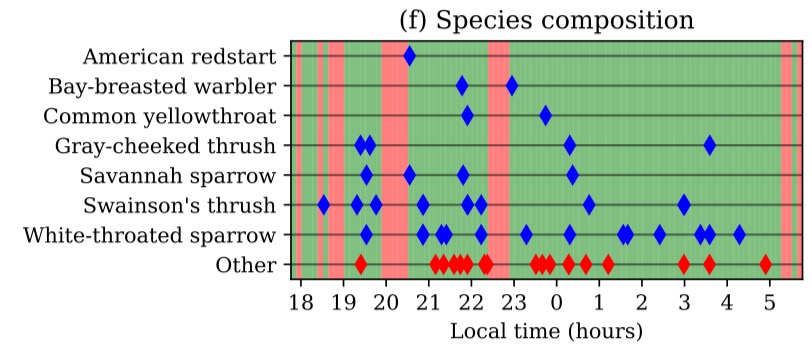
BirdVoxDetect: Large-Scale Detection and Classification of Flight Calls for Bird Migration Monitoring @ IEEE TASLP
Sound event classification has the potential to advance our understanding of bird migration. Although it is long known that migratory species have a vocal signature of their own, previous work on automatic flight call classification has been limited in robustness and scope: e.g., covering few recording sites, short acquisition segments, and simplified biological taxonomies. In this paper, we present BirdVoxDetect (BVD), the first full-fledged solution to bird migration monitoring from acoustic sensor network data.
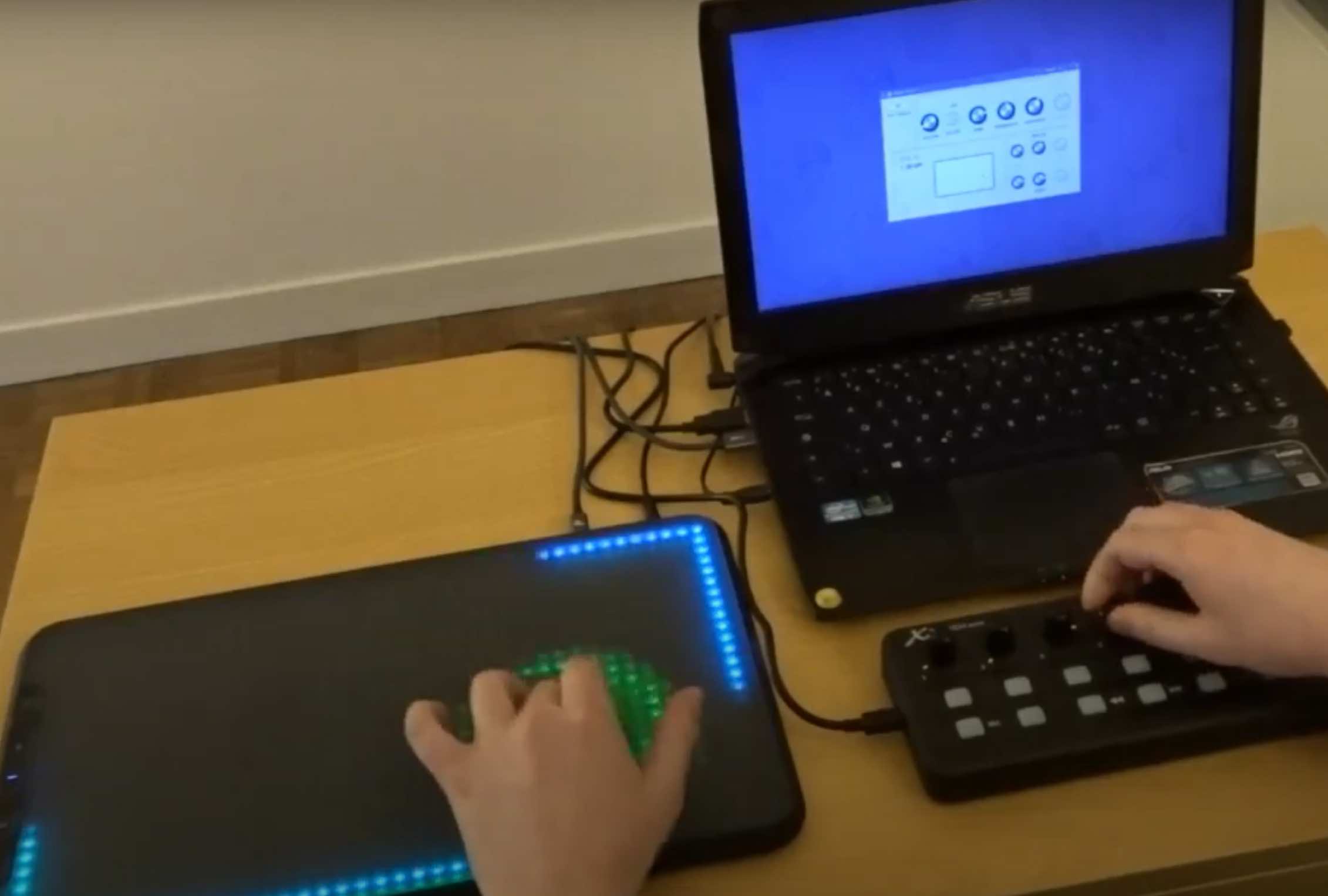
Loïc demonstrates his FTM Synthesizer on Erae Touch
A demonstration by independent researcher Loïc Jankowiak, who visited LS2N in 2023 and 2024 as part of the ApEx project of Han Han.
This is an in-progress build of a physically-modelled drum synthesizer using the Functional Transformation Method (FTM), with external MIDI control. The Embodme Erae Touch control surface is used for the velocity and impact position on the virtual drum’s surface, and the Behringer X-Touch Mini is used for controlling the various parameters of the physical model, i.e. the material properties.
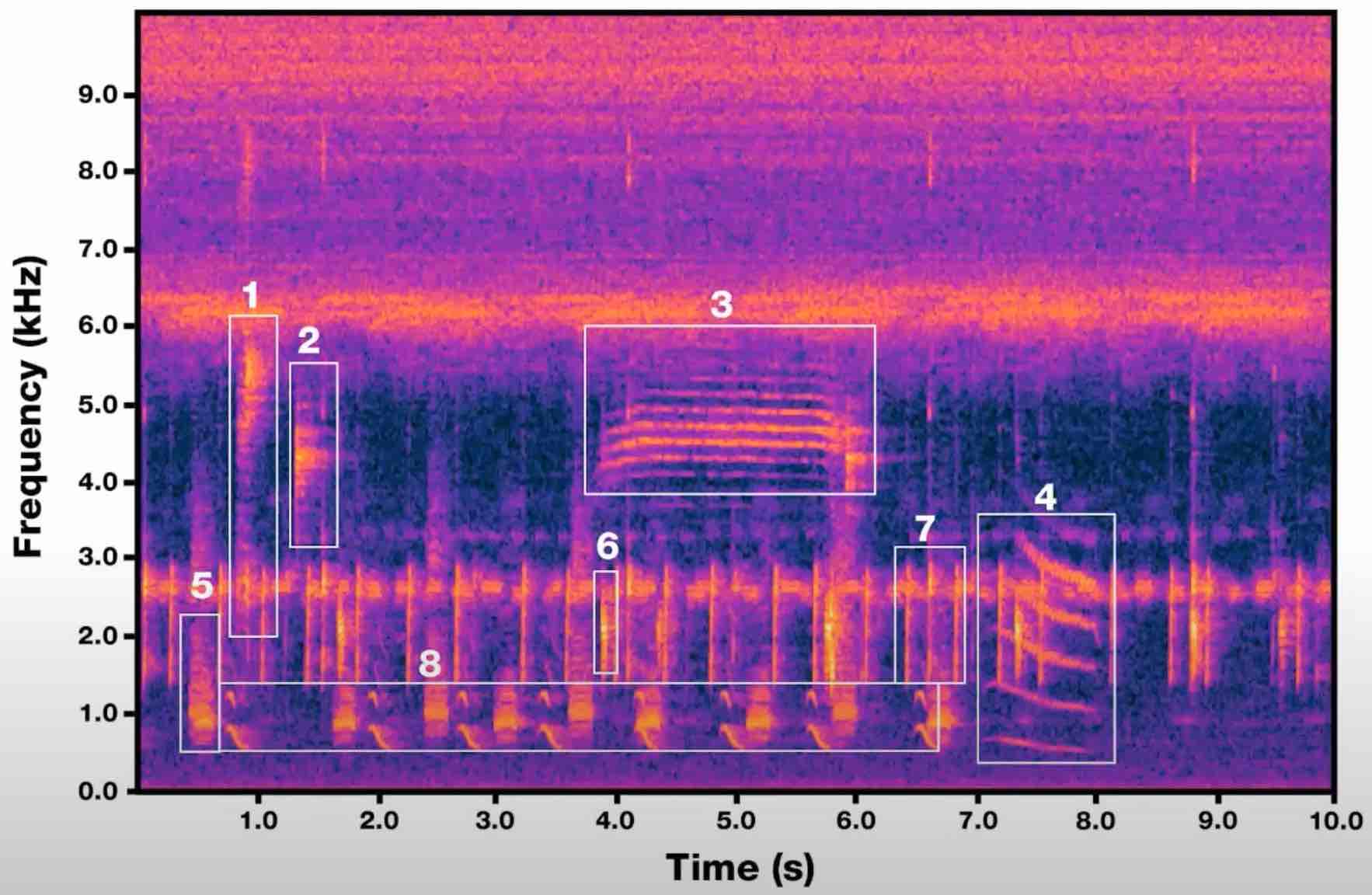
Mixture of Mixups for Multi-label Classification of Rare Anuran Sounds @ EUSIPCO
Multi-label imbalanced classification poses a significant challenge in machine learning, particularly evident in bioacoustics where animal sounds often co-occur, and certain sounds are much less frequent than others. This paper focuses on the specific case of classifying anuran species sounds using the dataset AnuraSet, that contains both class imbalance and multi-label examples. To address these challenges, we introduce Mixture of Mixups (Mix2), a framework that leverages mixing regularization methods Mixup, Manifold Mixup, and MultiMix. Experimental results show that these methods, individually, may lead to suboptimal results; however, when applied randomly, with one selected at each training iteration, they prove effective in addressing the mentioned challenges, particularly for rare classes with few occurrences. Further analysis reveals that the model trained using Mix2 is also proficient in classifying sounds across various levels of class co-occurrences.