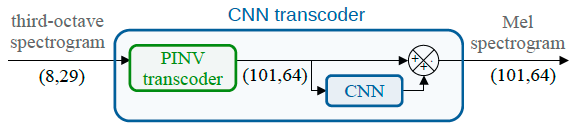
Spectral trancoder : using pretrained urban sound classifiers on undersampled spectral representations
Communications dans un congrès
Auteurs : Modan Tailleur, Mathieu Lagrange, Pierre Aumond, Vincent Tourre.
Conférence : 8th Workshop on Detection and Classification of Acoustic Scenes and Events (DCASE)
Date de publication : 2023
Convolutional Neural Network CNNGenerative algorithmThird-octave spectrogramMel spectrogramUrban soundscape